The ACLU of Maine works on a variety of issues in the Maine State Legislature to protect and expand the civil rights and liberties of all people in the Pine Tree State.
All of our work is done through a racial justice lens. Our nation's political system was designed to uphold white supremacy and built on the oppression of Black, Brown, and Indigenous people. We must dismantle the systems and policies that continue to uphold white supremacy and seek justice for past harms. Our team works closely with grassroots organizations and groups throughout Maine so the policies we promote at the State House reflect the realities of the people they will affect.
We just wrapped up the Second Regular Session of the 131st Legislature. The session ran from January 3 to May 10, 2024.
The Maine State Legislature operates on a biennial schedule, meaning the legislative process is divided into two regular sessions during each two-year cycle. The 131st Legislature started in December 2022 and ended on May 10, 2024.
The two-year cycle begins when the legislature convenes at the start of an odd-numbered year. The legislative session that occurs during the odd-numbered year is called the First Regular Session. The legislative session that happens in the second year is called the Second Regular Session.
Alongside our allies, members, and other advocates, the ACLU of Maine won a number of victories during the 2024 legislative session, from protecting criminal legal reforms to building momentum on data privacy. Bills referenced throughout this update are referred to as "LD," the abbreviation for "Legislative Document."
![]() |
![]() |
![]() |
![]() |
![]() |
![]() |
![]() |
![]() |
![]() |
Corporations have built a surveillance economy, collecting people’s most sensitive information without their consent – all to turn a profit. This risks our safety, exacerbates inequality, and threatens our democracy.
Following Maine’s leadership on data privacy in recent years, Big Tech flooded the State House with corporate lobbyists to oppose what could have become the nation’s strongest digital privacy law. They also pushed their own bill, LD 1973. Under the guise of protecting Maine people’s privacy, the industry bill would have allowed tech giants to continue exploiting our most personal information so long as they let us know in their privacy policy.
Alternatively, the bill that we championed, LD 1977, would have implemented sensible guardrails for companies operating in Maine. This groundbreaking legislation passed the House and fell just two votes short in the Senate. Most bills take years to pass. Getting a comprehensive bill like LD 1977 this close to the finish line in the first year built momentum.
Read more about how tech giants exploit our most personal information to exacerbate racial inequities, threaten reproductive freedom, and risk LGBTQ people’s safety here.
Return to 2024 Legislative Priorities and Victories.
Maine is one of just nine states without specific timelines in law to ensure that criminal cases proceed fairly and efficiently.
As a result, Maine is failing to uphold the people’s right to a speedy trial by burdening people accused of crimes with unprecedented delays, forcing them to wait months and even years for trial, often while incarcerated.
Despite this being the first year lawmakers considered the legislation, it passed both chambers. Unfortunately, LD 1771 did not receive funding and was not passed off the appropriations table, one of the last steps before heading to the governor’s desk.
Return to 2024 Legislative Priorities and Victories.
Maine will join the National Popular Vote Interstate Compact thanks to LD 1578. This bill seeks to ensure the presidential candidate with the most votes in the country wins — not most of the time, but every time.
Under the compact, states would give their electoral votes to the candidate for president who receives the most votes in the country. A national popular vote would ensure that each vote cast has an equal impact on the outcome of the presidential election, furthering the principle of one person, one vote.
When enough states join that they collectively hold 270 electoral votes (the amount needed to win the Electoral College), these states will give their Electoral College votes to the presidential candidate who wins the popular vote.
The Electoral College was created in large part to prop up the power of southern states as they disenfranchised enslaved Black people. Electing the president with the popular vote is one way to address our nation's harms and uphold the principle of “one person, one vote.”
Opponents of the law have filed paperwork for a people’s veto and are gathering signatures. If they collect enough signatures, the law's fate will go to Maine voters this fall.
Return to 2024 Legislative Priorities and Victories.
We defeated new attempts to double down on the failed and racist policies of the War on Drugs alongside other Maine advocates. Lawmakers must focus on root causes of problematic drug use: poor access to health care, housing, jobs, and education. Incarceration will never solve a public health crisis.
For instance, LD 2204 would have prohibited people from buying property based solely on their national origin, along with provisions that would increase incarceration rates and expand police surveillance without probable cause.
LD 2204 echoed repeated efforts over the past century to weaponize false claims of “national security” against Asian and other immigrants. It would have prohibited people from purchasing property in Maine if they are not permanent residents and are citizens of China, Cuba, Iran, North Korea, or Russia.
The bill targeted people based on something they cannot control – where they were born – and was not directed at specific conduct. Discrimination based on national origin, race, ethnicity, color, or alienage violates the Equal Protection Clause of the Fourteenth Amendment. Several similar laws have been reversed in many states over the past century. Earlier this year, a federal appeals court blocked a similar racist property ownership law in Florida in a challenge brought by the ACLU.
Return to 2024 Legislative Priorities and Victories.
Maine is the only state that relies almost entirely on private attorneys to provide legal representation to people who cannot afford their own attorney.
As a result, the state has violated the Sixth Amendment rights of people accused of a crime in Maine for decades. Lawmakers allocated roughly $4 million in LD 653 to create four new public defender offices in Maine, new rural public defender positions, and new staff for the central office for public defense. This legislation will fund four offices covering Aroostook, Penobscot, Piscataquis, Oxford, Franklin, Hancock and Washington counties.
While this funding is a step in the right direction, our lawsuit against the state for failing to uphold people's Sixth Amendment right to effective assistance of counsel continues. As of this writing, we are preparing to return to trial. We have expanded our initial lawsuit against the state to address the ways the system has deteriorated since we first sued in 2022. Read more about this case, Robbins v. MCILS, here.
Moreover, the number of people denied their Sixth Amendment right to an attorney has skyrocketed, growing 497% from November 2023 to May 2024.
Our recent analysis of state data shows hundreds of people are currently being denied their right to counsel, and many of those people are incarcerated at the same time. Additionally, counties with the higher rates of people denied the right to counsel typically have higher prosecution rates in general. See the full report here.
Return to 2024 Legislative Priorities and Victories.
The answer to Maine’s deadly overdose epidemic will never be punishment and incarceration, and decades of “tough on crime” policies have proven it.
While LD 1975, legislation to approach this issue holistically and through a public health lens, did not pass, the legislature allocated funding for at least two receiving centers in Lewiston and Aroostook County by passing LD 1714.
People with substance use disorder need low-barrier access to community-based treatment. Punishment and incarceration will never help people work through a health issue. Instead, the failed policies of the War on Drugs have played a significant role in creating the current crisis by directing resources to criminal legal policies that tear families and communities apart.
Read more about the true cost of the failed War on Drugs and a better path for Maine in our report co-authored by the ACLU of Maine and Maine Center for Economic Policy here.
Return to 2024 Legislative Priorities and Victories.
LD 2001 would have given teachers and schools the necessary resources to comply with a 2001 law requiring schools teach Wabanaki and African American Studies.
This legislation initially passed both chambers with bipartisan support, but it was not funded by the end of the legislation session and failed to make it to the governor’s desk.
As a state and country, we must learn the full context of the past and present so we can make informed decisions about the future. This includes understanding the colonization that led to the creation of the place we now call Maine, and the genocide, oppression, and attempted erasure of the Wabanaki. It also includes the role of many prominent Mainers in the slave trade, and the many contributions that Wabanaki and African American people have made and continue to make to this place that we all call home.
This was the first year this legislation won initial passage in both chambers. We will continue working with our coalition partners to ensure Maine’s teachers have the resources and guidance they need to teach our state’s complete history.
Return to 2024 Legislative Priorities and Victories.
LD 1642 made important updates in the language used to discuss the Wabanaki nations, people, and systems of government.
This new law changed terms such as “Maine Native American Studies” to “Wabanaki Studies.” Our laws must respect and reflect the reality of the people they concern by using accurate and considerate language.
Return to 2024 Legislative Priorities and Victories.
The original version of LD 2046 would have made permanent a law allowing the state to incarcerate legally innocent people instead of providing necessary medical care. Maine should not send legally innocent people to prison, for healthcare or for any other reason.
Since 2013, Maine law has allowed the Department of Health and Human Services to transfer people who have been ruled incompetent to stand trial to the Intensive Mental Health Unit at the Maine State Prison. This was not intended to be a permanent solution.
This policy was set to expire on July 1, 2024, but lawmakers attempted to extend the practice indefinitely. Following close work with lawmakers, we successfully persuaded the Senate to set an expiration date of July 1, 2027, and the House concurred. The final legislation will also convene a group to study ways the state can provide behavioral health care outside of a prison setting.
If Maine has the capacity to care for people with acute mental illness in a prison setting, then the state has the capacity to do the same outside of a prison setting. While this practice should not occur at all, it was crucial for the legislature to set a clear end date for this harmful policy.
Return to 2024 Legislative Priorities and Victories.
2025 will be the First Regular Session of the 132nd Legislature. We plan to build on our momentum to push some of this year's priorities over the finish line. We also anticipate lawmakers will introduce many bills to restrict Maine people's civil rights and liberties.
During the first session of each two-year cycle, legislation covers a broad range of topics because lawmakers are free to submit as many bills as they please and on any topic. During the Second Regular Session, lawmakers can only consider bills related to the budget, presented by the governor, or approved by the Legislative Council.
As happened in 2023 during the first session of the 131st legislature, we are expecting many bills in 2025 designed to undermine Maine people's civil rights and liberties. This legislation will likely include attacks on voting rights, LGBTQ equality, reproductive freedom, and immigrants' rights. We also expect attempts to reverse our criminal legal reform wins over the past five years. We will continue to bring the fight with our coalition partners to defend and expand the civil rights and civil liberties of all people in Maine.
2024 is an election year and all Maine lawmakers are on the ballot.
Know your rights when voting, make a plan to vote, and spread the word at our Voter Information Center.
Date
Wednesday, May 22, 2024 - 11:15amFeatured image
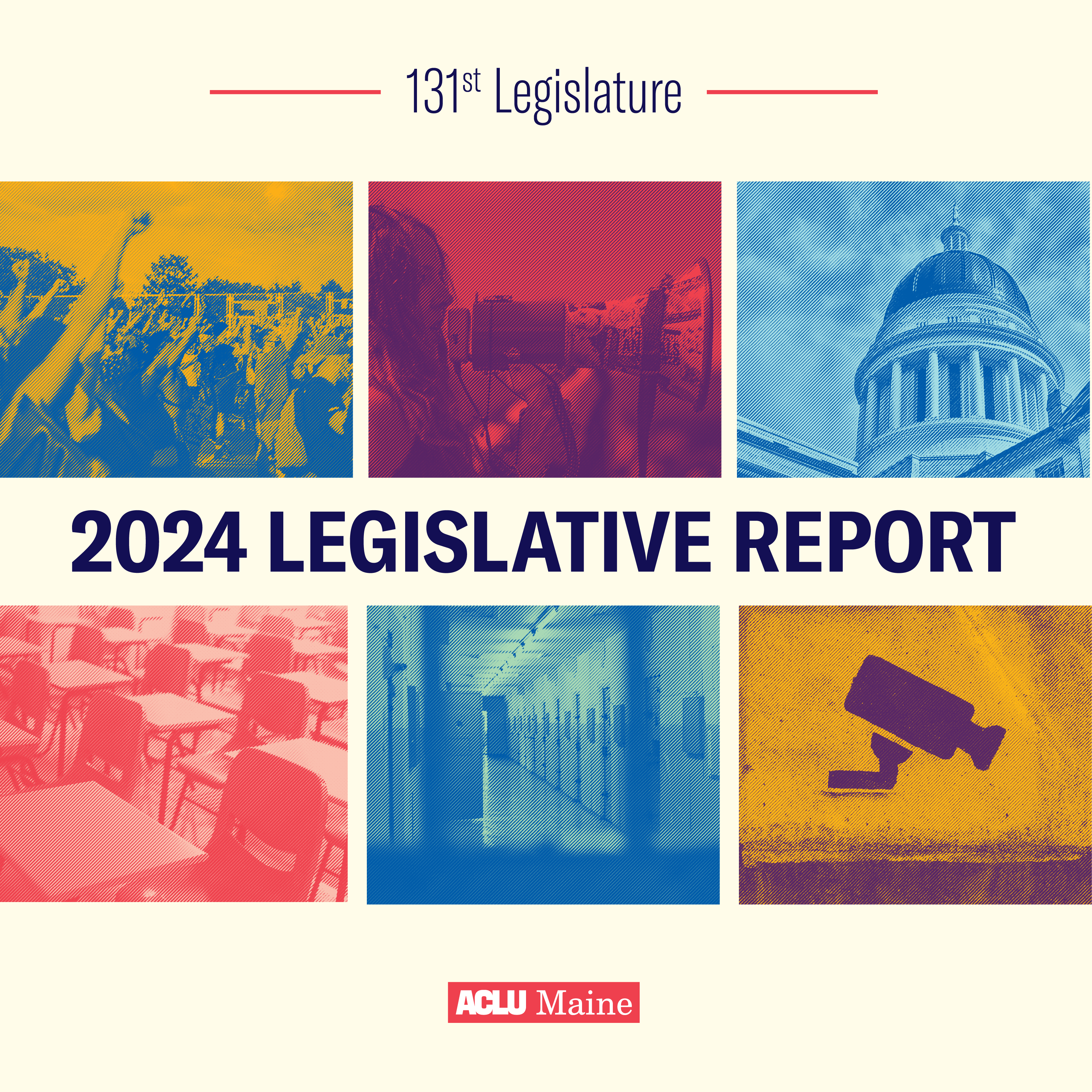